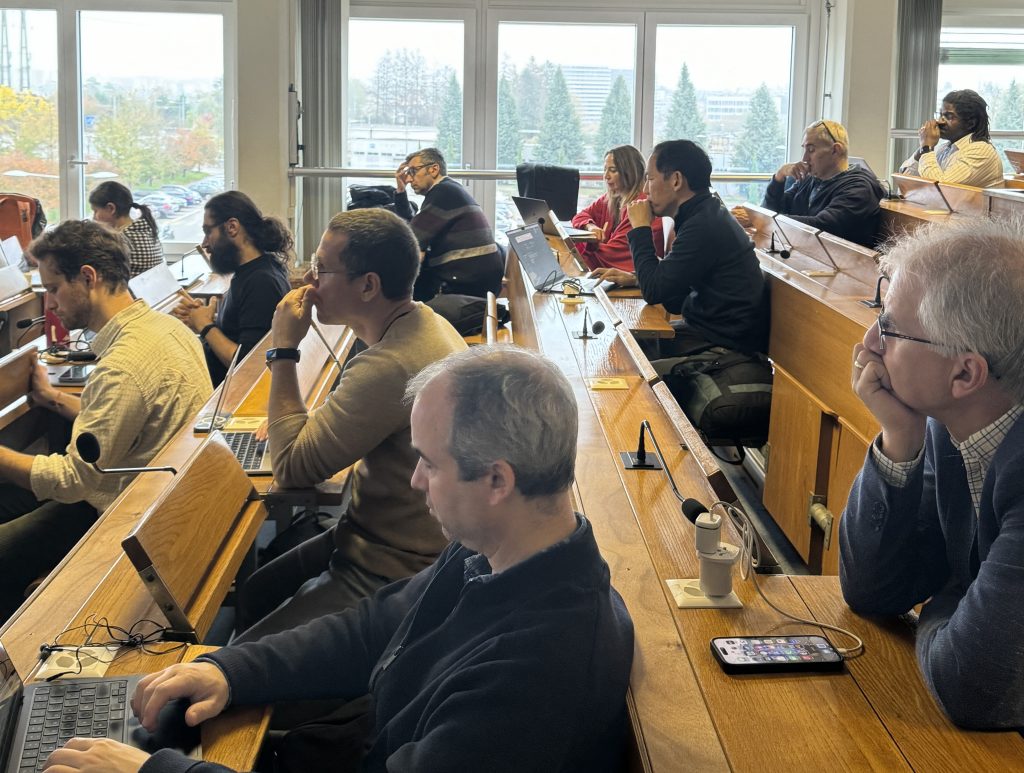
As scientific discovery in high-energy physics reaches unprecedented depths, the computational challenges of analyzing vast datasets and complex interactions are becoming increasingly daunting. On November 4-5, 2024, CERN hosted the Workshop on Tensor Networks and (Quantum) Machine Learning for High-Energy Physics, an important event in the journey of the Next Generation Triggers (NGT) project. Organized under Task 1.4, this workshop gathered experts to discuss how advanced computational methods, specifically tensor networks (TN) and quantum machine learning (QML), can address the data complexities of high-energy physics and drive more efficient data processing for next-generation experiments.
With participants from leading institutions, including CERN, the University of Padova, and several international universities, the two-day event fostered collaboration and explored the latest theoretical and practical advancements that could revolutionize the analysis pipeline for particle physics.
The Intersection of Quantum and Classical Computing
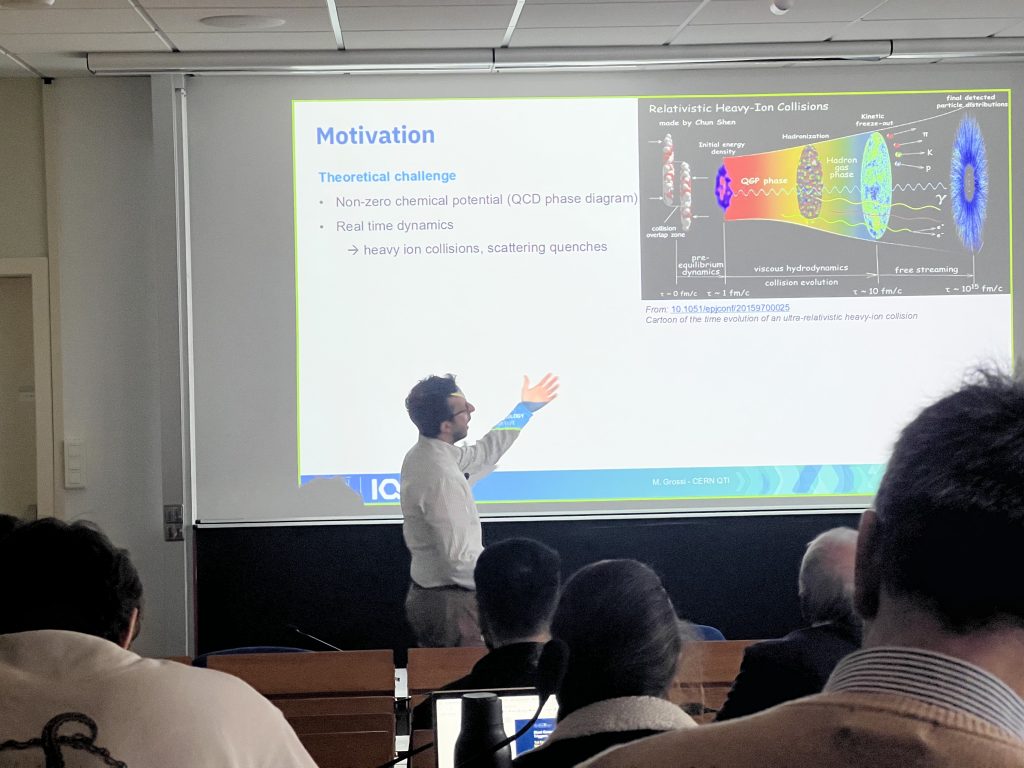
Quantum machine learning combines quantum computing’s raw processing potential with machine learning’s pattern-recognition prowess, creating possibilities for breakthroughs in processing and understanding HEP data.
Dr. Michele Grossi, from QTI, kicked off the event with a keynote on integrating QML into HEP data analysis pipelines, discussing how QML can enhance anomaly detection, event classification, and cross-section integration, all critical tasks for analyzing particle collision data. Quantum-enhanced techniques hold promise for efficiently tackling these tasks by leveraging quantum bits (qubits) that process exponentially more data than classical bits.
However, the path forward is far from straightforward. Quantum algorithms require optimization and adaptation to handle the kinds of data and models used in HEP effectively. Tensor networks, originally developed for studying quantum systems, offer a framework that efficiently represents quantum states, making them highly relevant for high-energy physics research. Dr. Mari Carmen Bañuls shared insights on how tensor networks can represent equilibrium states in lattice gauge theories, a crucial tool for understanding fundamental interactions in particle physics.
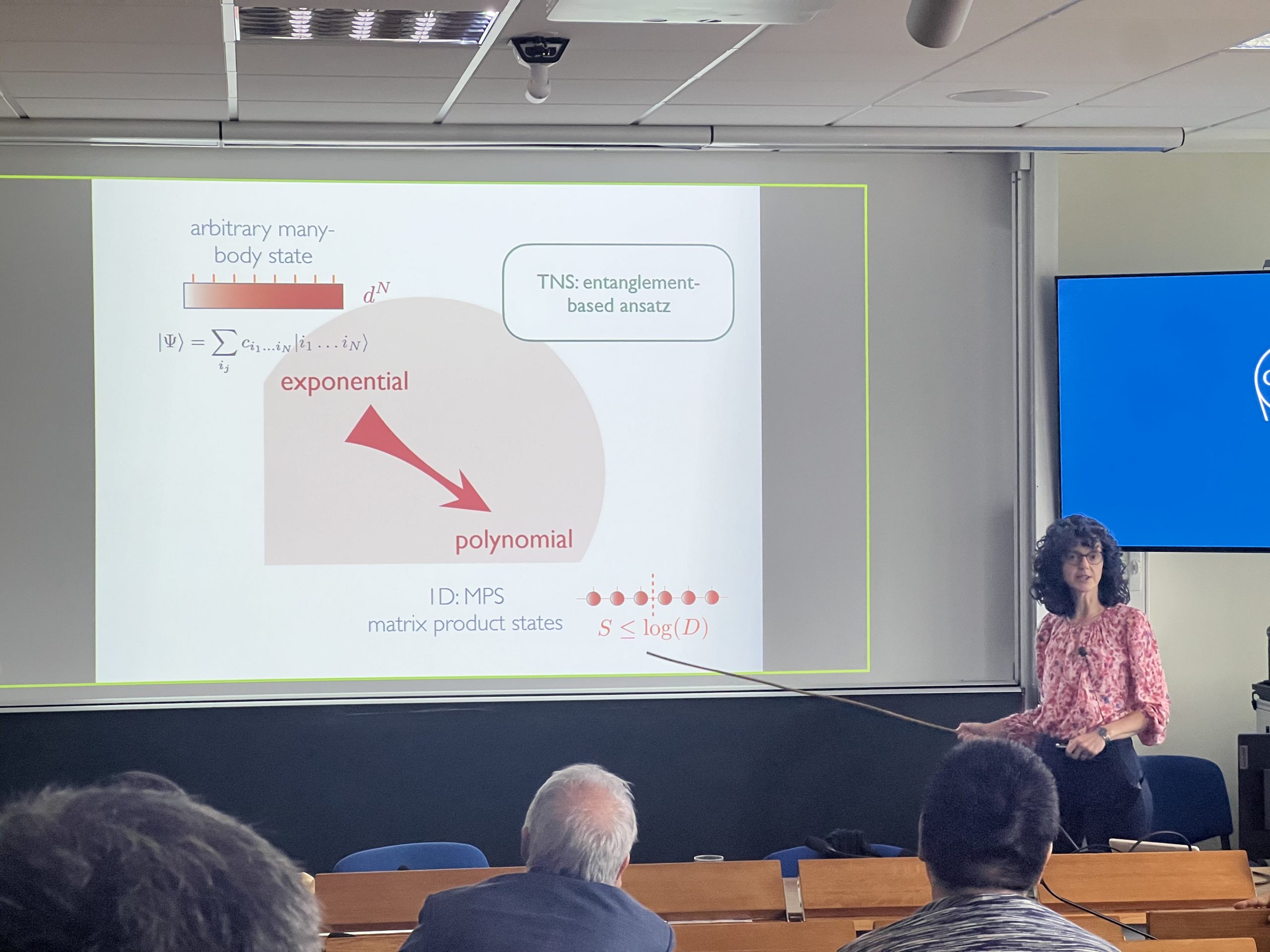
Tensor Networks: A New Way of Modeling Complex Systems
Tensor networks are mathematical structures used to represent and manipulate large amounts of information in complex systems, especially those with quantum characteristics. In high-energy physics, these networks allow scientists to simulate and predict quantum behaviors within the constraints of current computational power. For example, when studying lattice gauge theories—which model the fundamental interactions within particle physics—tensor networks offer a way to compress and organize data, making it possible to conduct simulations that would otherwise require prohibitively large computational resources.
Ors Legeza discussed recent developments in tensor network state methods that enhance nuclear physics applications, focusing on methods for modeling quantum many-body interactions with high accuracy. These methods, particularly useful in ab initio nuclear physics calculations, were presented as a way to refine models of phenomena like nuclear structure and particle interactions at scales that are impractical for traditional methods.

Simone Montangero further expanded on tensor network methodologies by presenting advancements in tree tensor network algorithms for quantum simulations. He highlighted their applications in lattice gauge theories in 2+1 and 3+1 dimensions at finite density and out-of-equilibrium scenarios in 1+1 dimensions. Montangero also outlined a roadmap for advancing tensor network simulations to handle increasing complexity, as well as their use in solving hard classical combinatorial problems. Additionally, he discussed tensor network machine learning applications, such as b-bbar tagging at LHCb, showcasing the versatility of these techniques for both theoretical and experimental challenges.
Real-Time Applications and Hardware Innovations
The workshop also highlighted the practical challenges and opportunities of implementing tensor networks and QML in real-time applications. Hardware limitations remain a significant hurdle in deploying QML in HEP, as high-frequency environments such as online triggers in particle detectors demand rapid and precise calculations. Alberto Coppi and Lorenzo Borella shared pioneering work on optimizing tensor network algorithms for deployment on high-performance GPUs and FPGAs, enabling the concurrent operations essential for real-time HEP data processing.
Additionally, Elias Fernandez-Combarro brought insights from a computer science and engineering perspective, describing strategies to overcome hardware limitations and integrate tensor networks into high-energy physics infrastructure effectively.

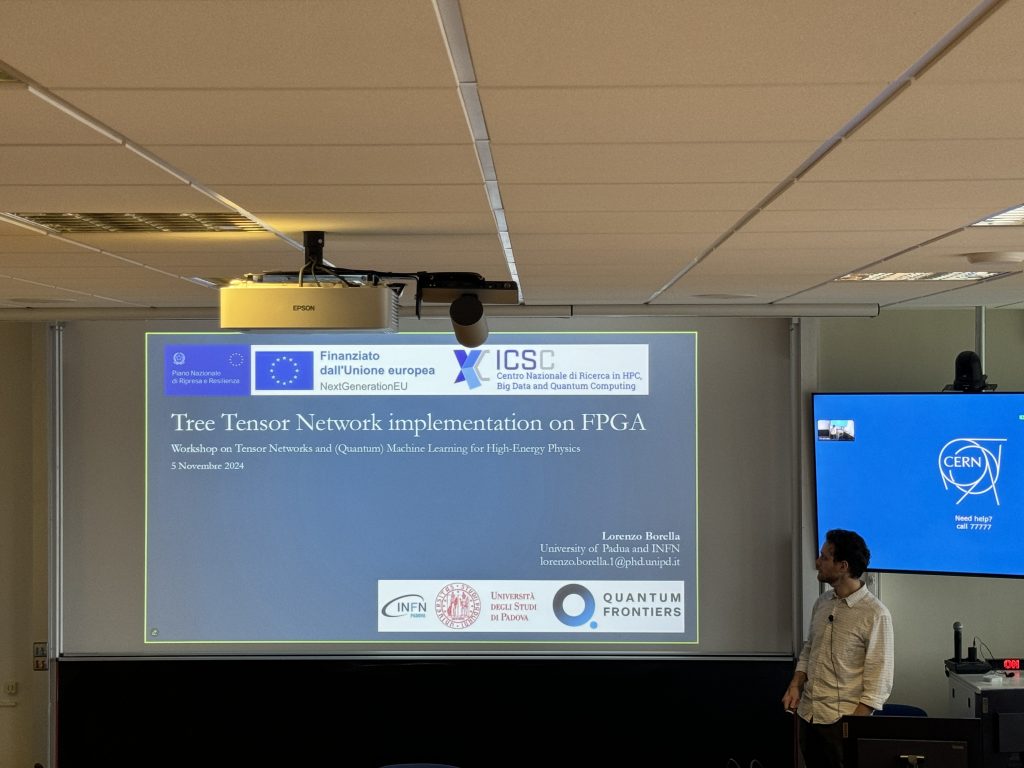
Provable Advantages and Future Prospects
A particularly exciting aspect of the discussions was the potential for provable quantum advantages in data processing. Although many theoretical and practical obstacles remain, researchers are beginning to identify scenarios where QML algorithms could outperform classical methods exponentially. Dr. Vedran Dunjko presented theoretical advancements demonstrating quantum machine learning’s potential for exponential speedups over classical approaches in specific learning tasks. By applying quantum learning algorithms to HEP’s data-rich environment, researchers may soon achieve advantages in tasks like anomaly detection and event classification—two crucial areas in particle physics data analysis.

for quantum-generated data”. Picture: Alex Lasa Lamarca
This prospect of quantum advantage underscores the importance of interdisciplinary research, where physics, computer science, and engineering come together. As Dr. Dunjko noted, future breakthroughs in HEP may rely on innovations that transcend traditional disciplinary boundaries, allowing us to harness quantum mechanics’ full potential to reveal new insights into the universe’s fundamental building blocks.
Collaboration for the Future of High-Energy Physics
The workshop’s collaborative atmosphere highlighted the importance of bringing together diverse expertise and perspectives. With discussions ranging from the theoretical underpinnings of tensor networks to hands-on implementation strategies, the event demonstrated the necessity of a shared vision to advance HEP.
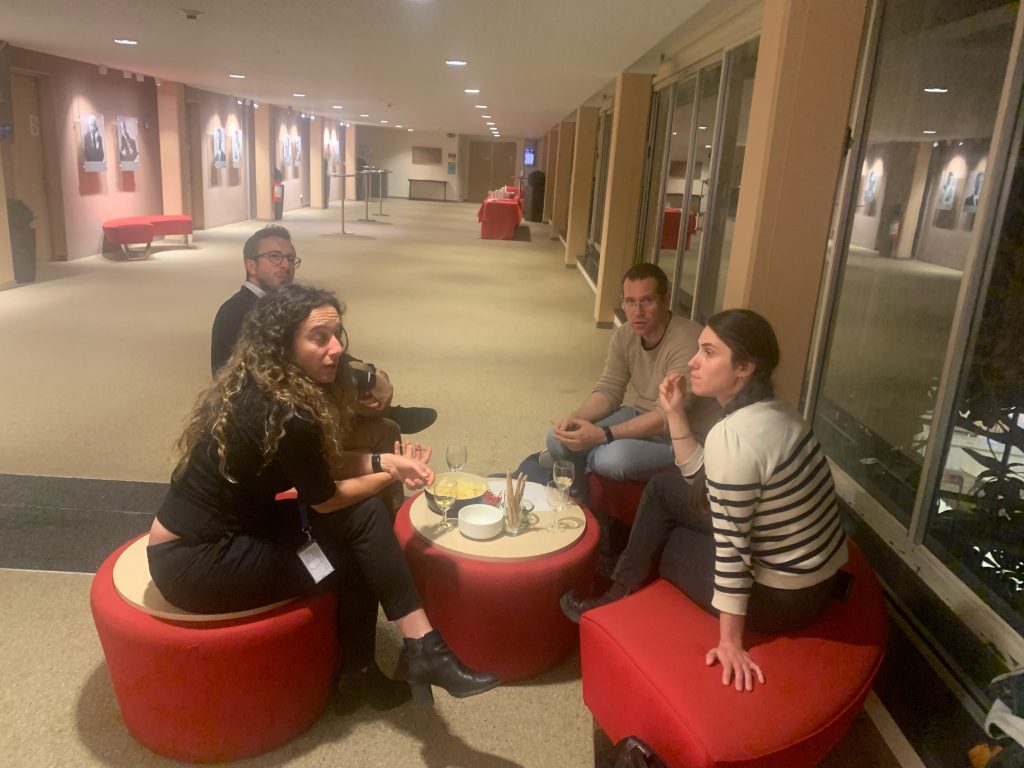

As the Next Generation Triggers project advances, workshops like this are pivotal in shaping the future of high-energy physics. These events provide a platform for researchers to explore innovative computational approaches that push the boundaries of what is scientifically possible. As Enrique Ortega Rico, Task 1.4 leader, emphasized: “By delving into tensor networks and quantum machine learning, researchers are establishing a foundation for transformative breakthroughs.” These breakthroughs, he noted, “aim to address some of the most profound and challenging questions in physics—questions that test the boundaries of our understanding of the universe and redefine the capabilities of classical and quantum computation.”