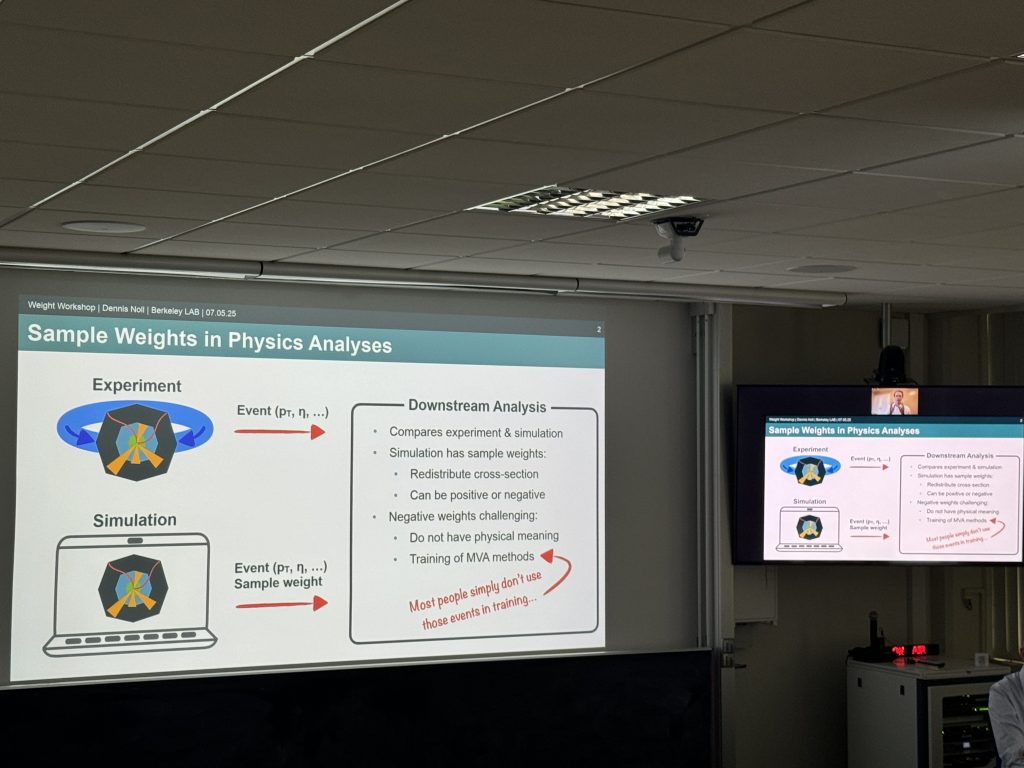
Last week, from May 5 to 8, researchers from across the high-energy physics community gathered at CERN to take on a thorny problem in modern event generation: negative weights.
As computing demands rise with the High-Luminosity LHC (HL-LHC), so too does the urgency to make Monte Carlo (MC) simulations more efficient. Negative weights—an artefact of next-to-leading-order (NLO) and higher-order calculations—dilute statistical power and inflate computational costs. This workshop, hosted under Task 1.5 of the Next Generation Triggers (NGT) project, brought together theorists and developers to exchange ideas and explore innovative strategies to mitigate this issue.
The format included short, focused talks paired with plenty of discussion time, allowing participants to dig deeper into proposed solutions and coordinate ongoing efforts.
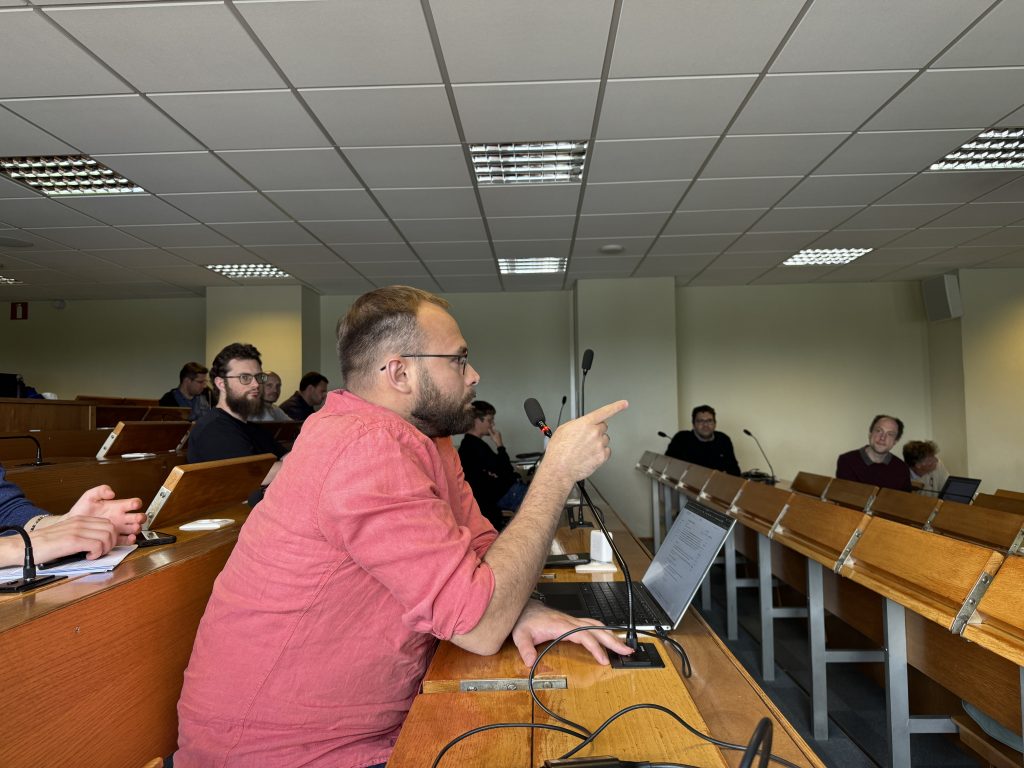
Among the key highlights, participants explored several promising strategies. KrkNLO Matching and ESME (Exponentiated Subtraction for Matching Events) presented approaches that generate positive-weight samples by construction, avoiding subtraction techniques that commonly lead to inefficiencies. ARCANE Reweighting introduced a novel Monte Carlo reweighting scheme capable of drastically reducing negative weights without altering the underlying matching frameworks.
Discussions also covered challenges in NNLO QCD calculations, with insights from the MATRIX framework and developments like cell resampling aimed at improving statistical efficiency. On the machine learning front, innovative applications of Normalising Flows and neural weight refinement techniques showcased how modern AI tools can help reshape simulation strategies for high-dimensional event data.
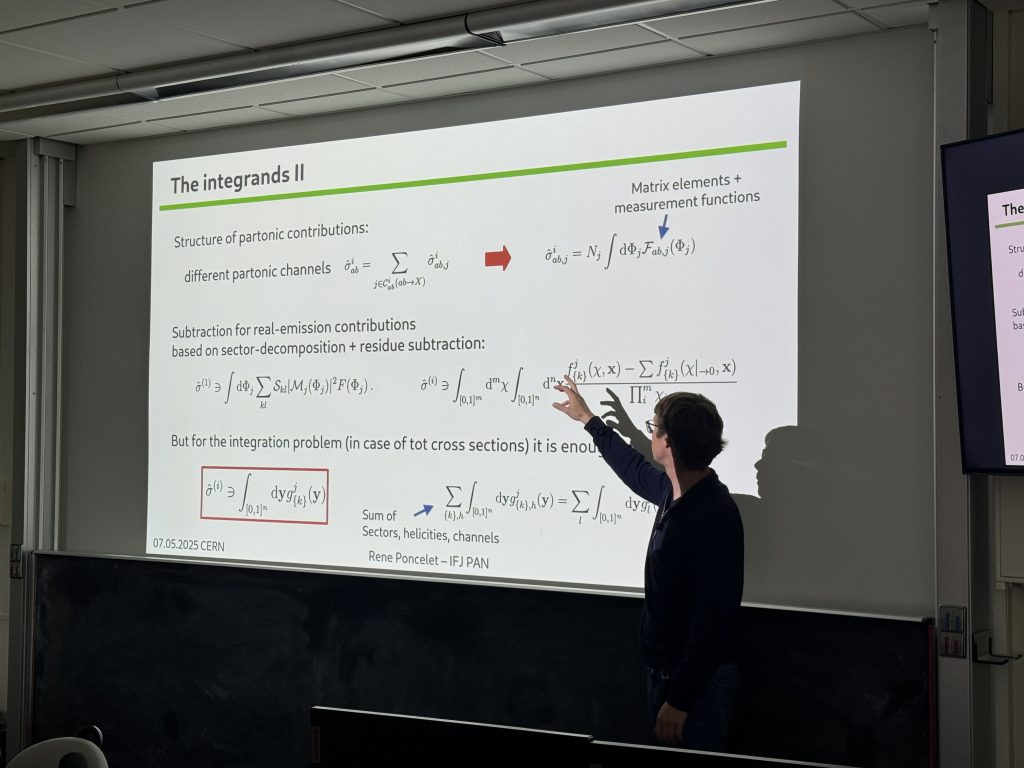
If you are interested to know more, you can check out all the presentations and contributions from the event by clicking here.